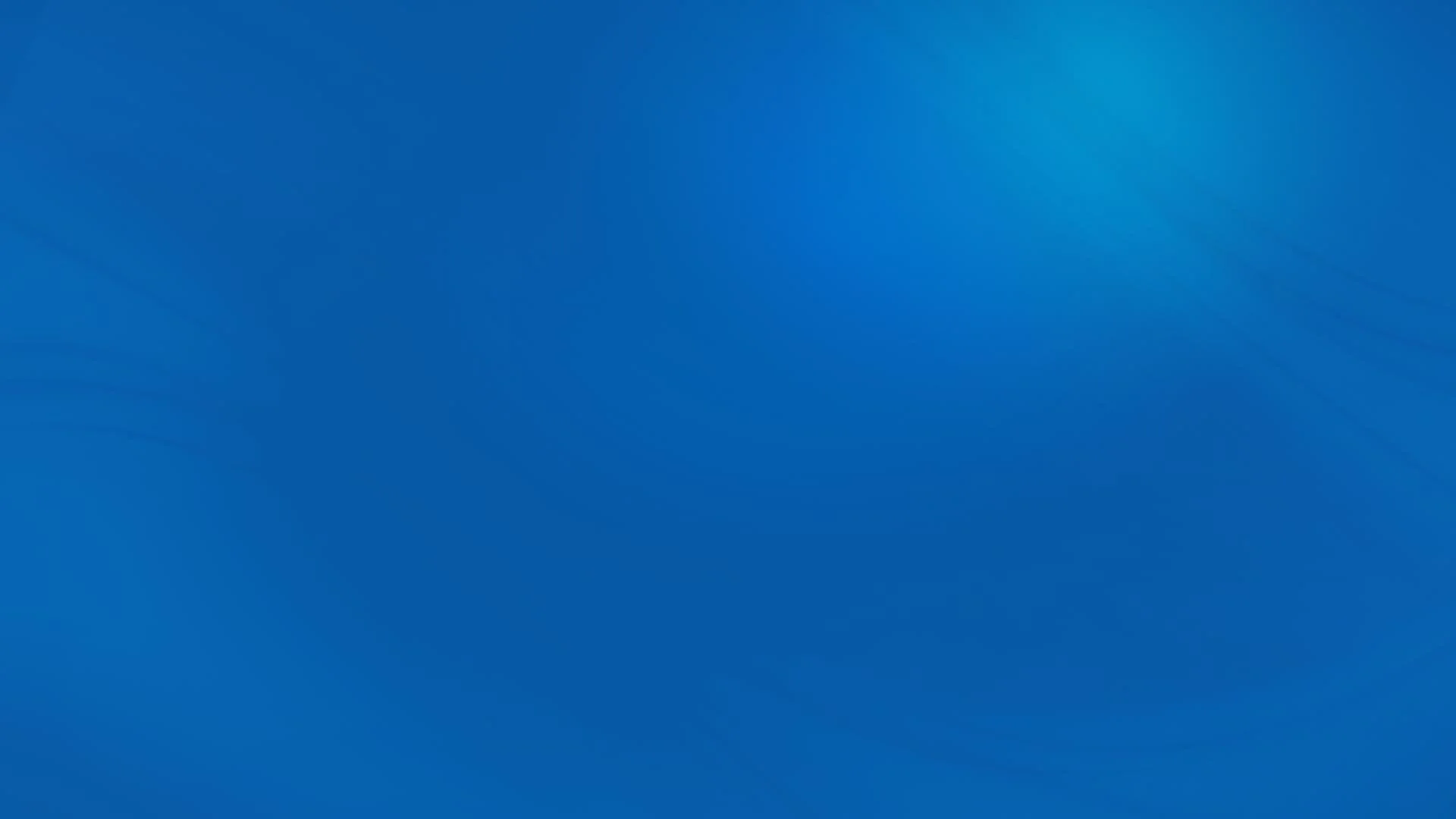
See the latest updates from our team, and all the projects we’re working on.

Part 2: Siloed Documentation in a Collaborative World
The clinical note has been a cornerstone of medical documentation for decades. While this structure may have worked in the past, the note paradigm is increasingly out of place in today’s healthcare environment. In this post, we’ll explore how notes — organized around visits and not around problems— create information chaos in healthcare and why we need to move toward a new documentation model.

The Future of Live Documentation - Addressing the Growing Problem of Medical Documentation Overload
As AI continues to transform healthcare, many assume it can fix the growing issue of documentation overload. While AI offers just-in-time summaries and automation, relying solely on it without improving how data is structured leads to bloated, disorganized charts. In our latest post, we explore why better organization—through problem-oriented documentation and structured data—is key to streamlining workflows, reducing costs, and enhancing patient care.
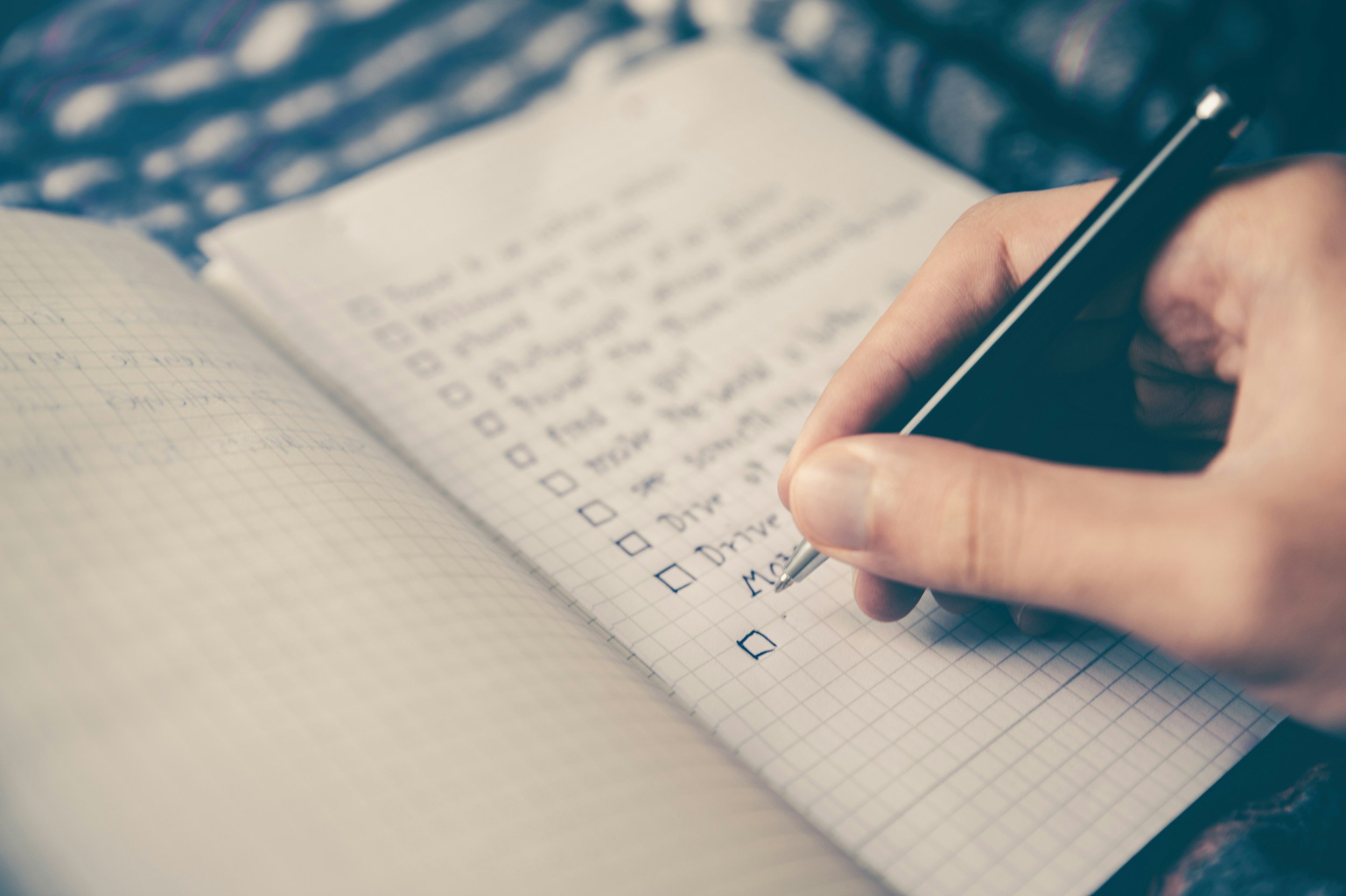
The Danger of Pre-Templated Information in Medical Records
Templating notes, exams, care plans, and histories can be bad for patient care, even if it's good for clinician efficiency. Clinical documentation ought to accurately reflect the hard work clinicians put into their care. Fortunately, large language models can help build better documentation that is reflective of the vibrancy of the patients they describe.

Part 1: The Note, A Relic of the Paper Era
A brief history of the medical note and how it has come to frustrate clinicians and patients across the world.
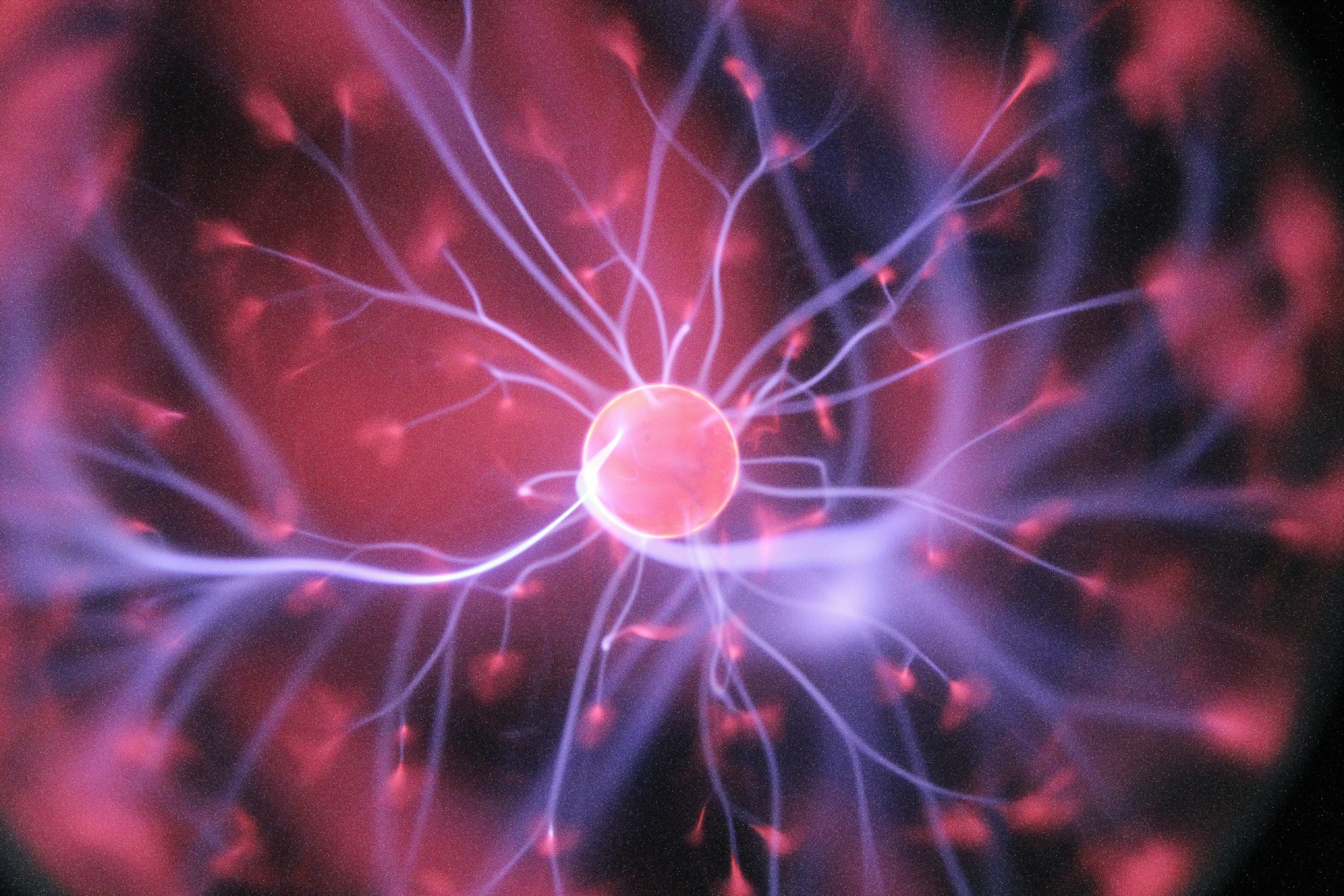
Redefining Ambient Clinical Intelligence
Written by: Alex Butler, MD, MS - Pediatrician & Chief of Product
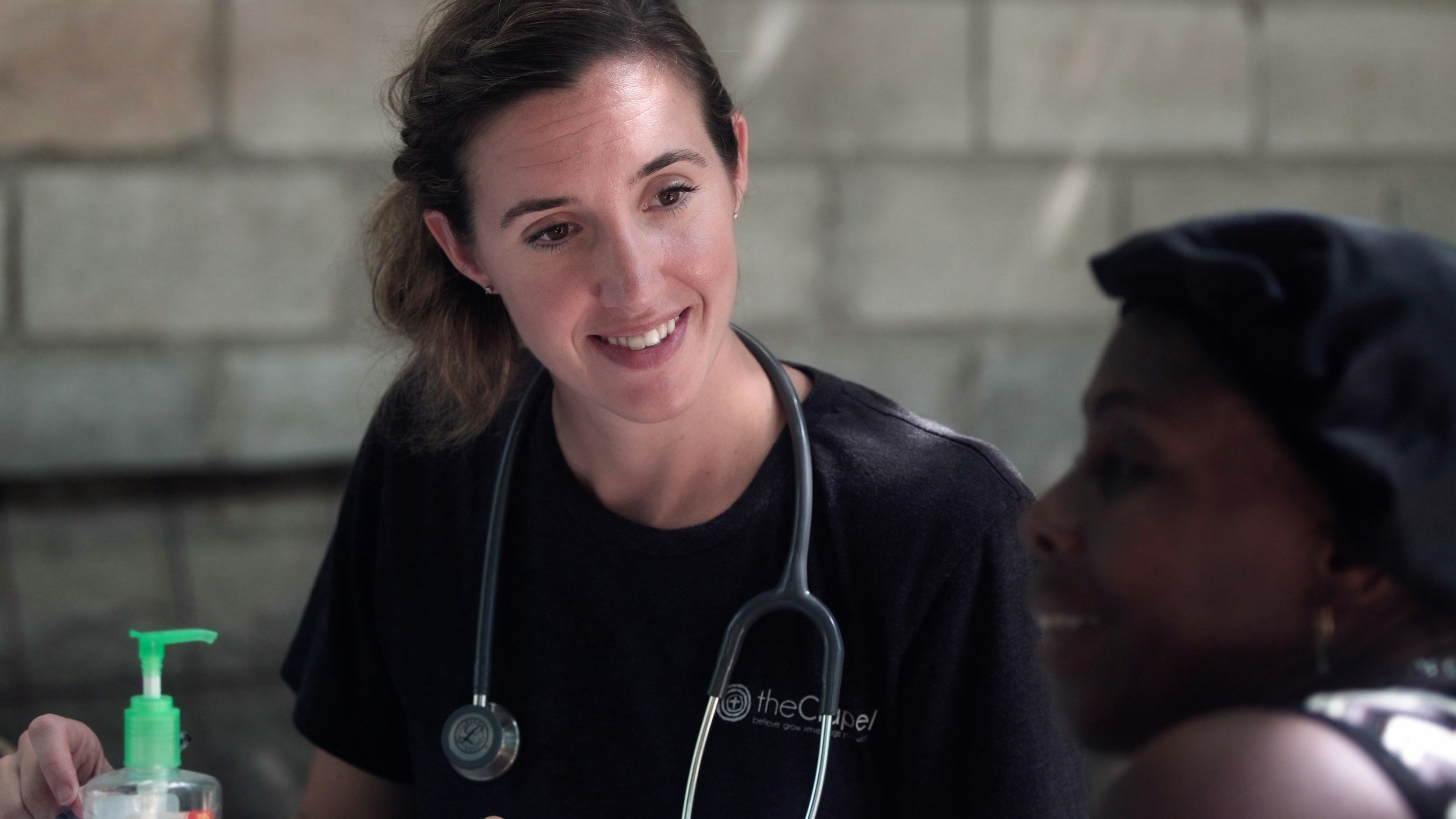
Talk to your Patients, not the Computer
Written by: Abhinav Sharma, MD - Primary Care Physician & CEO
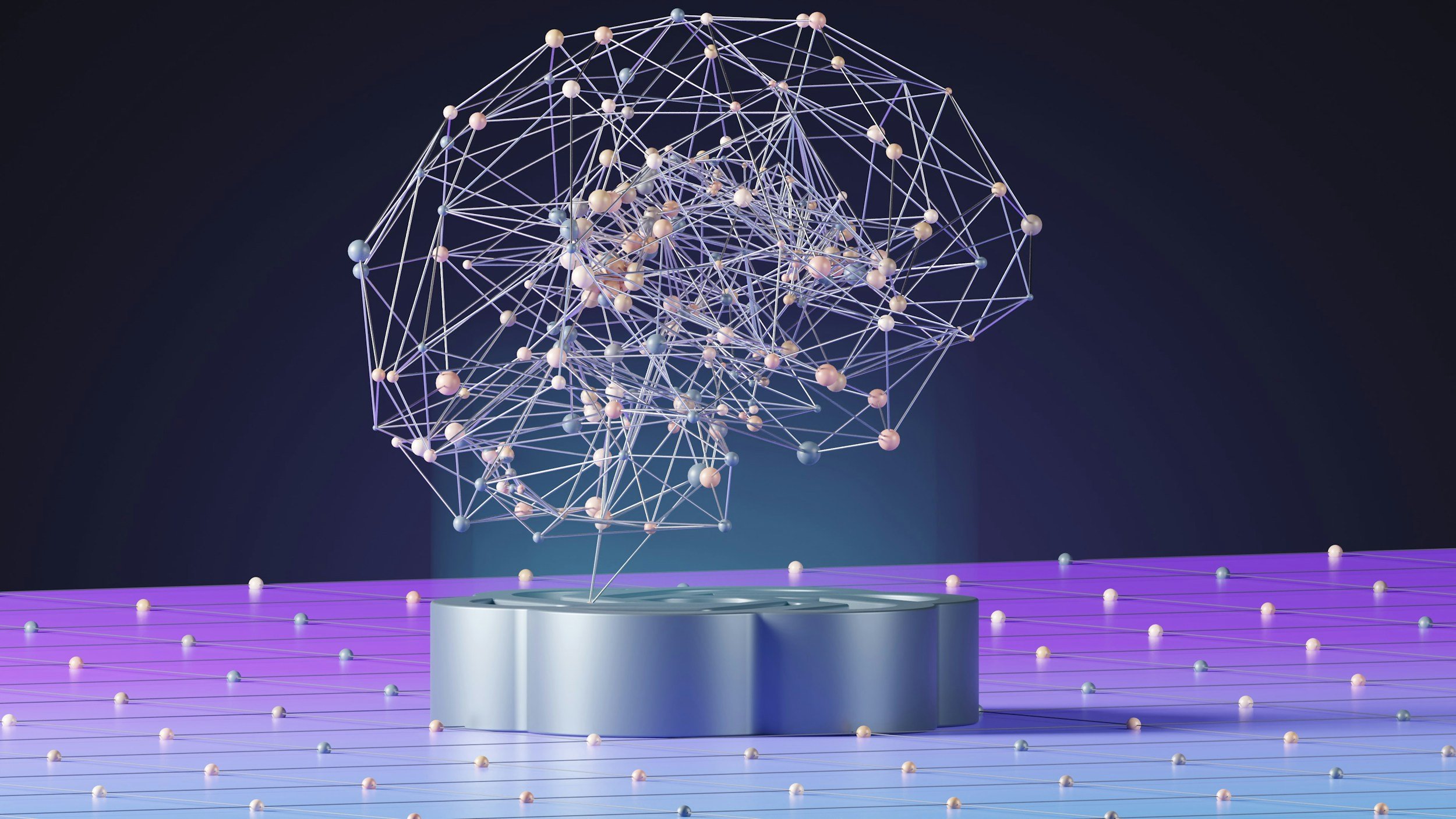
“I suppose it is tempting, if the only tool you have is a hammer, to treat everything as if it were a nail.”
Written by: Derrick Chu, MD - Acute Care Pediatrician & VP of Product
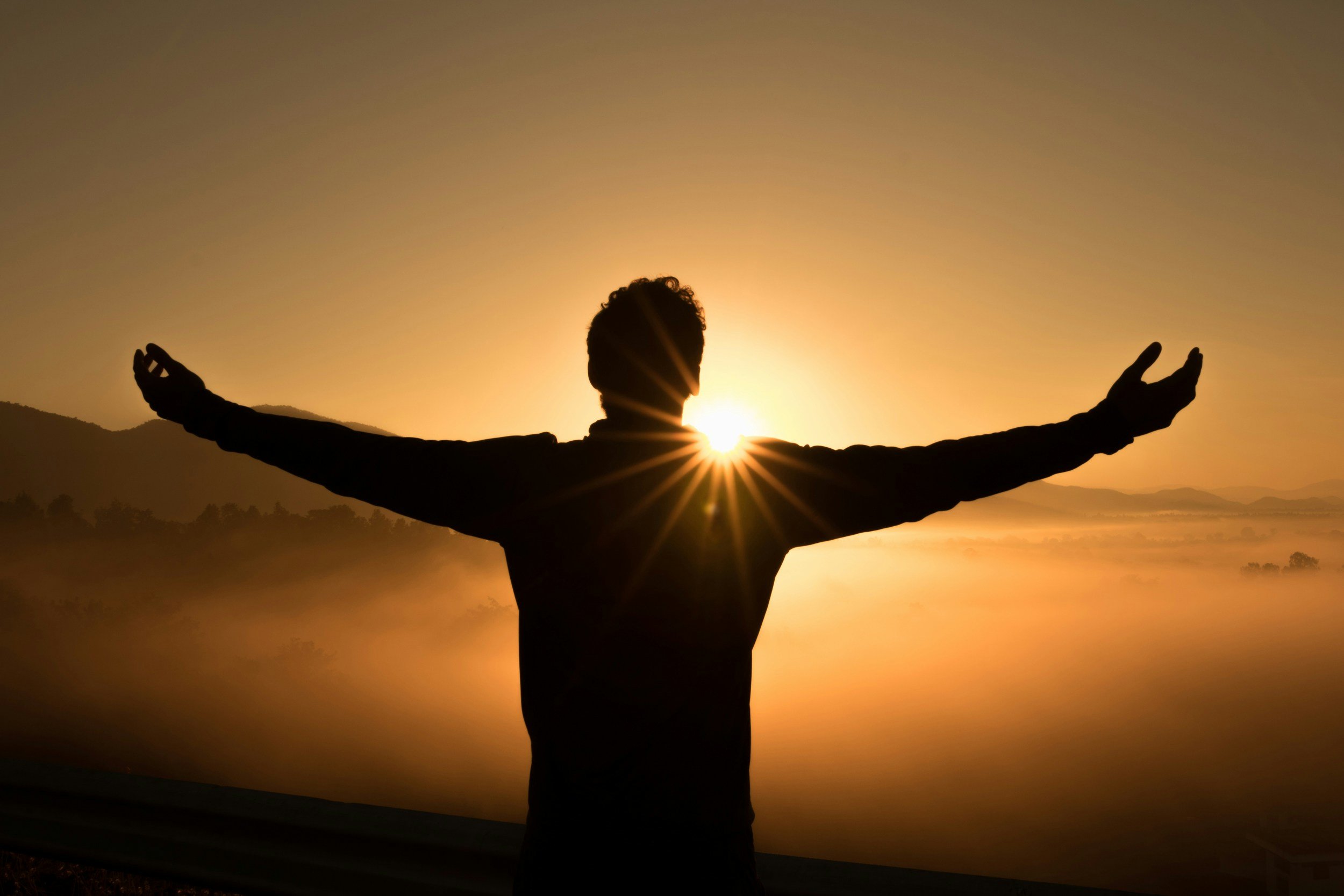
Finding Balance? Trying to Navigate Clinician Wellness
Written by: Ariana Meltzer-Bruhn, MD - Pediatrician
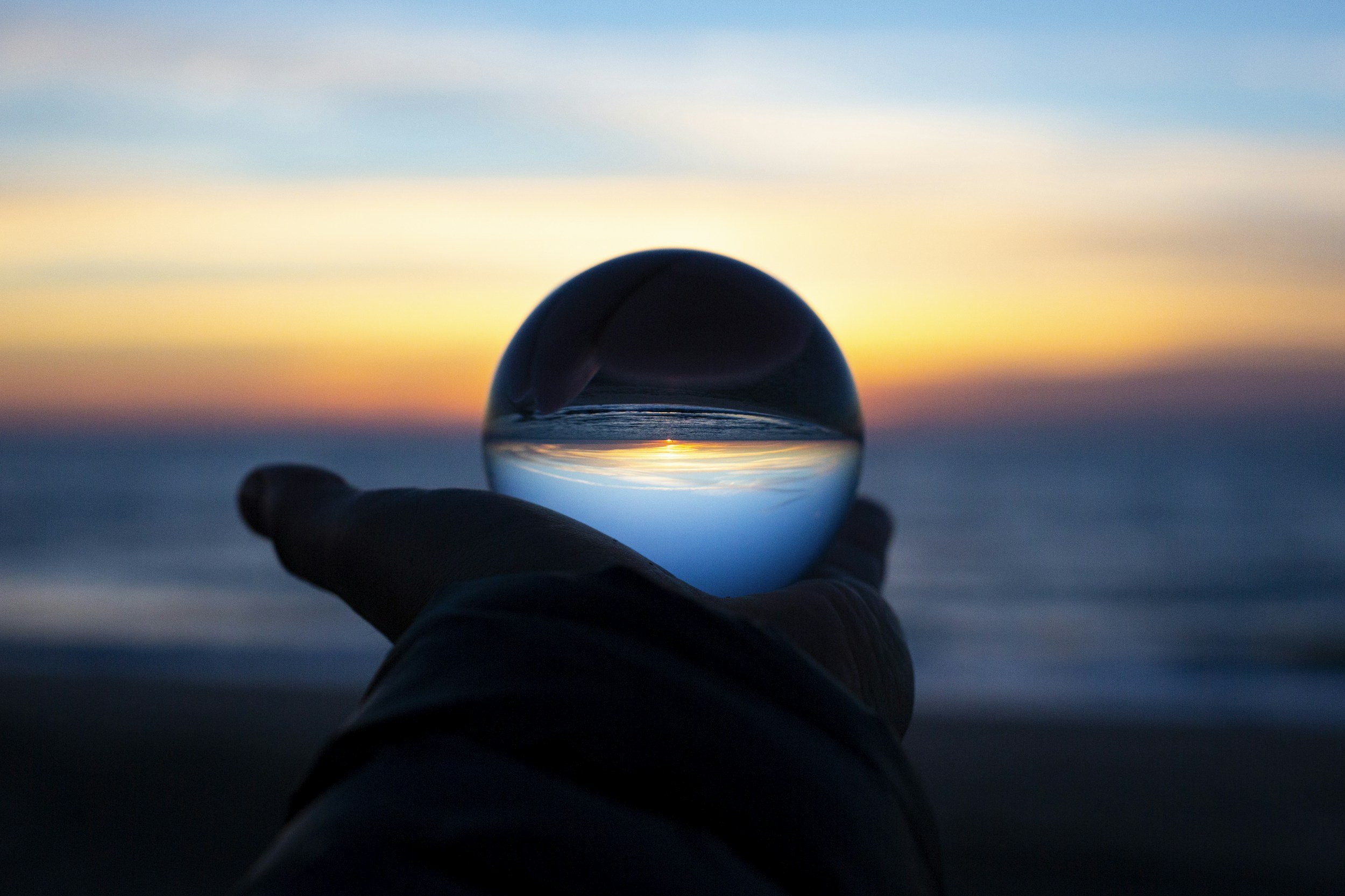
Where We’ve Been and Where We’re Going
Written by: Jacob Kantrowitz, MD, PhD - Primary Care Physician & Chief Medical Officer
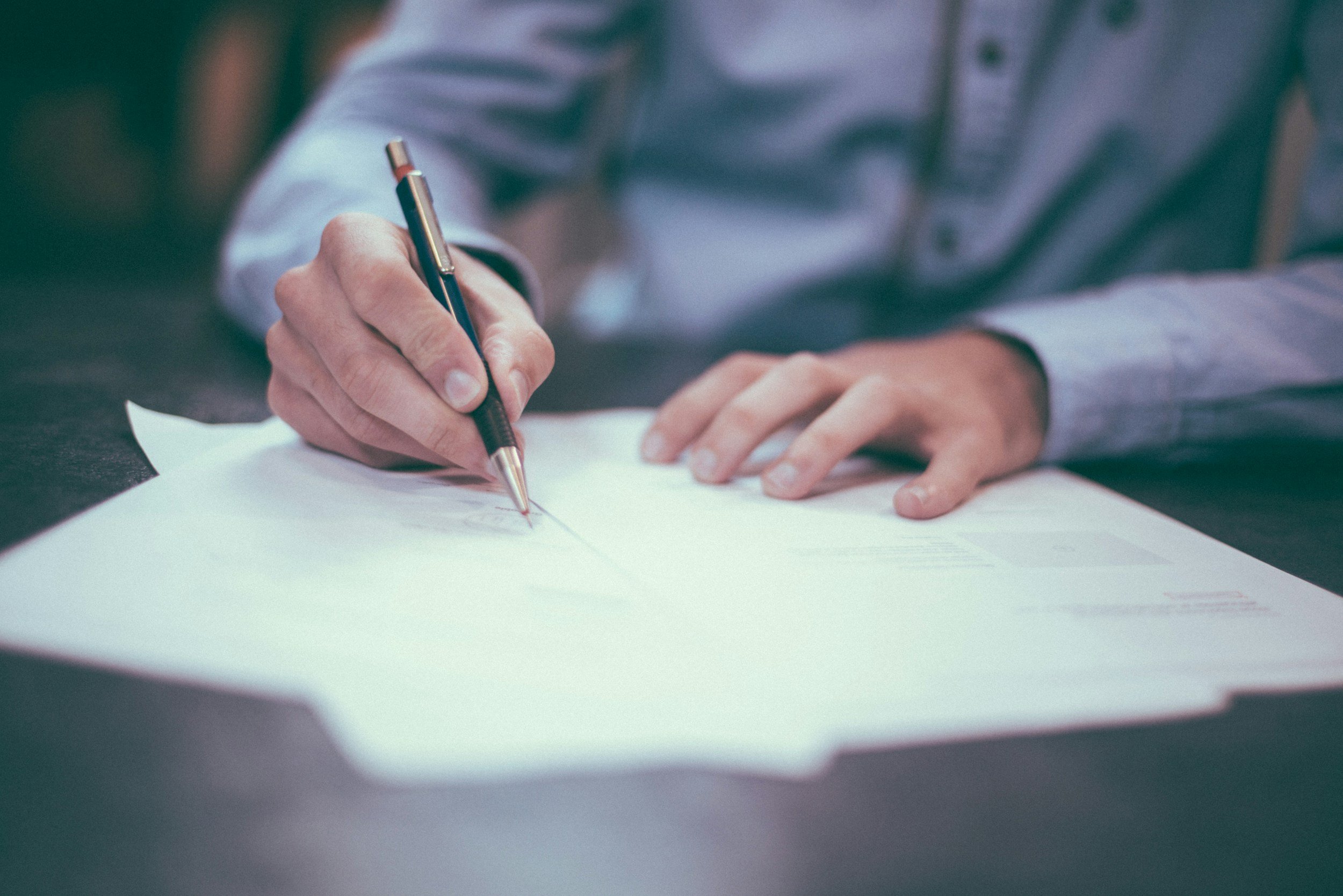
Clinical Notes – Not the Revolution We Were Promised
Written by: Alex Butler, MD, MS - Pediatrician & Chief of Product
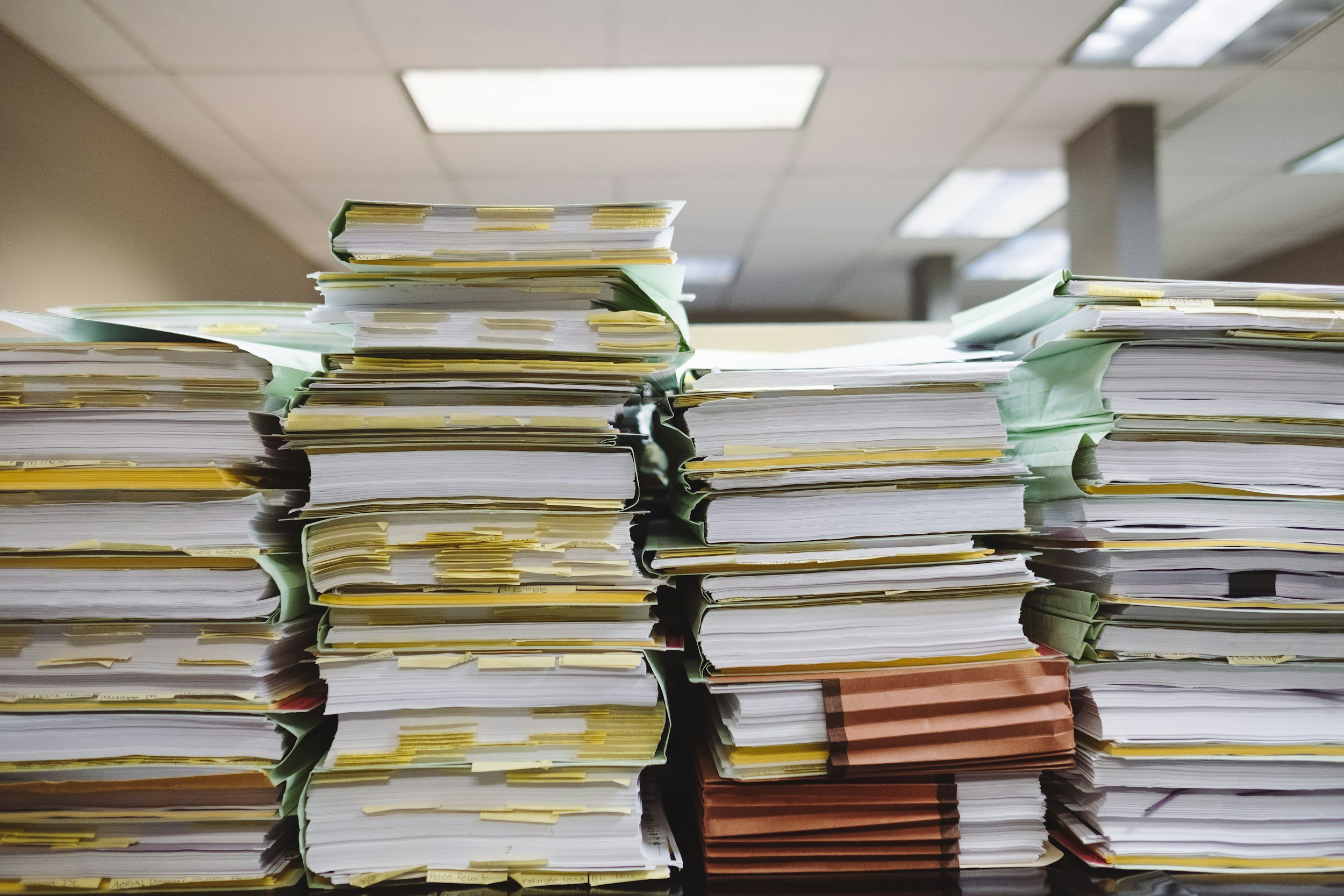
Documentation Sucks: How did we get here?!
Written by: Abhinav Sharma, MD - Primary Care Physician & CEO

Prevalence and Sources of Duplicate Information in the Electronic Medical Record
The prevalence of information duplication (copy-paste) in electronic medical records (EMRs) suggests that it is an adaptive behavior requiring further investigation so that improved documentation systems can be developed.

A Fully Collaborative, Noteless Electronic Medical Record Designed to Minimize Information Chaos: Software Design and Feasibility Study
We propose and build a prototype of the world's first noteless electronic health record, which is built to optimize collaboration and minimize information chaos.

Beyond Notes: Why It Is Time to Abandon an Outdated Documentation Paradigm
The medical chart—including notes, labs, and imaging results—should be reconceptualized as a dynamic, fully collaborative workspace organized by topic rather than time, writer, or data type. This will lead to better clinical outcomes and higher job satisfaction among clinicians, who will suffer less with decreased cognitive burden.

A Web Application for Adrenal Incidentaloma Identification, Tracking, and Management Using Machine Learning
Incidental findings are a common medical problem that are prone to falling through the cracks of the medical system. Building safety net systems to identify, track, and to help manage these potentially dangerous findings can decrease the cognitive burden on physicians and lead to better outcomes for patients. In this manuscript, we present a software system designed to identify adrenal incidentalomas and track them over time.

Task definition, annotated dataset, and supervised natural language processing models for symptom extraction from unstructured clinical notes
We present a clinically motivated task definition, dataset, and simple supervised natural language processing models to demonstrate the feasibility of building clinically applicable information extraction tools